How Alternative Data and AI/ML Models will Revolutionize Credit Risk Assessment in Brazil?
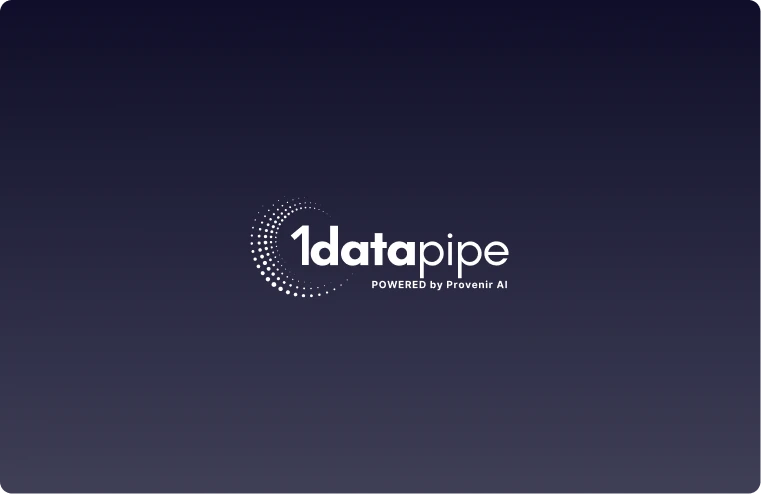
In Brazil, as in other emerging economies, there is a significant portion of the population that lacks a traditional credit history. This gap in data can often result in inaccurate risk profiling, leading to missed opportunities for both financial institutions and the individuals they serve. This has prompted financial institutions to seek alternative means of assessing credit risk.
One of these alternatives involves studying credit behavior patterns using alternative data. This data includes payment histories from utility bills, credit cards, retail cards, loans, and even past inquiries with other credit bureaus, along with information about bounced checks and collections.
Examining this array of data can provide a comprehensive view of a person’s creditworthiness. For instance, regular and timely payments of utility bills or rent can suggest a level of financial stability, while a history of bounced checks or multiple inquiries with credit bureaus might raise red flags.
Unlike traditional credit assessment methods, alternative data allows lenders to monitor an individual’s financial health continuously. This ongoing analysis reveals patterns that, when broken, can signal a heightened risk of default. By looking beyond the standard credit file and capturing a more holistic view of an individual’s financial behavior, lenders can make more accurate decisions and mitigate potential losses.
Affluence Indicators and Credit Risk
Moreover, assessing credit risk is not only about understanding financial behaviors. It also involves understanding the lifestyle and economic stability of an individual. Hence, affluence indicators such as assets owned, job titles, current employment and employment history, education, income, and job stability also play a vital role.
For example, a stable job, higher education, or significant assets can indicate a lower risk of default. Similarly, changes in job titles or employment status can provide real-time insights into an individual’s financial situation, which could directly impact their ability to fulfill credit obligations.
Layered Risk Approach: Factoring Fraud Risk into Credit Risk
When assessing credit risk, it’s essential to consider the associated fraud risk. A layered risk approach ensures a comprehensive risk analysis that goes beyond creditworthiness and includes potential fraud indicators.
This approach involves deploying sophisticated AI/ML models that can identify unusual patterns or behaviors that may signify fraudulent activity. For example, sudden changes in spending behavior, multiple credit inquiries in a short period, or inconsistencies in personal information can all be indicators of potential fraud.
By incorporating fraud risk into the overall credit risk assessment, financial institutions can further enhance their risk management strategies and ensure they’re lending responsibly.
Predictive Analysis and Real-Time Credit Risk Assessment
With the advent of machine learning and artificial intelligence, predictive analysis has become a critical tool for financial institutions. ML/AI models can utilize alternative data to predict credit risk in real-time, allowing financial institutions to quickly adapt to changes in a customer’s financial health.
In Brazil, despite efforts from fintechs like Nubank and Banco Inter to incorporate AI/ML models into their credit risk assessment processes, challenges remain. These companies have started to leverage big data and sophisticated algorithms to predict future payment behavior based on a client’s past transactions and other relevant financial information.
However, even with advanced AI/ML models, reaching the entire population, especially those without traditional credit history, remains a significant challenge.
The Limitations of Current AI/ML Models
While AI/ML models hold immense potential, their success relies on the quality of the data inputted into them. Traditional data sources often overlook the unbanked or underbanked population, creating a blind spot in the credit assessment process. This issue persists, even with the use of AI/ML models, because these technologies cannot create insights where data does not exist.
Moreover, traditional models often cannot handle the complexity and speed of modern financial transactions. This means they might miss critical signals, resulting in inaccurate risk assessment and potential losses for financial institutions.
Navigating the Future of Credit Risk Assessment in Brazil
Given the limitations of traditional credit assessment methods, there is a growing need for financial institutions in Brazil to adopt a more comprehensive, data-driven approach. By integrating alternative data and leveraging AI/ML models, lenders can better understand their customers’ credit behavior and make more accurate risk assessments.
Taking the Next Step with Next-Generation Credit Risk Insights
At 1datapipe, we understand the complexities of modern credit risk assessment. Our Living Identity Scores, backed by 370+ unique personalized attributes on customer profiles, provide an unprecedented level of detail on a customer’s credit behavior. With our real-time insights, financial institutions can better detect and prevent fraud, accurately assess credit risk, and promote financial inclusion among the underbanked population.
By harnessing the power of alternative data, 1datapipe enables financial institutions to gain a more comprehensive view of their customers’ creditworthiness. Contact us today to learn more about how we can help you enhance your credit risk assessment capabilities.