How AI Will Transform Credit Risk Models in Indonesia’s Embedded Finance Market
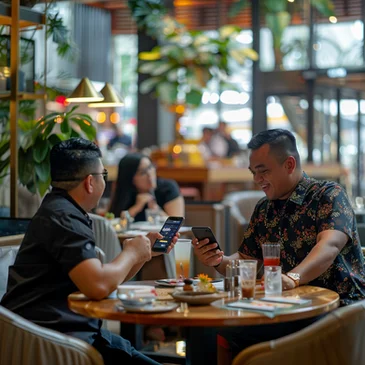
In the bustling streets of Jakarta, Rizky, a young entrepreneur, uses his smartphone to access a range of financial services seamlessly integrated into his favorite e-commerce platform. This effortless access to finance, whether for a small loan to boost his inventory or insurance for his motorbike, represents the future of financial services in Indonesia.
The rise of embedded finance, where financial products are seamlessly integrated into non-financial platforms, is revolutionizing how Indonesians interact with financial services. According to a recent report by ResearchAndMarkets.com, the embedded finance market in Indonesia is set to grow by 40.8% in 2024, reaching $2.59 billion, with projections to hit $10.54 billion by 2029.
While the explosive growth of embedded finance offers immense opportunities, it also presents significant challenges for risk executives at banks and fintech companies. To capitalize on this trend and stay ahead of the competition, these companies must go beyond merely embracing embedded finance. They need to harness a larger universe of alternative credit data and leverage AI-driven predictive consumer analytics models to make more informed credit risk decisions, particularly when expanding their customer reach to underserved populations and informal workers.
The Rise of Embedded Finance in Indonesia
Indonesia’s embedded finance market is driven by several key factors:
1. High Internet Penetration: With over 70% of the population connected to the internet, digital financial services have a broad and fertile ground to grow.
2. Large Unbanked Population: More than 74% of Indonesians are unbanked or underbanked, making them prime candidates for innovative financial solutions that embedded finance offers.
3. Supportive Regulatory Environment: The Indonesian government actively promotes financial inclusion and fintech innovation, creating a conducive environment for embedded finance to flourish.
4. Widespread Smartphone Use: With 80% smartphone penetration and over 212 million mobile internet users, access to embedded financial services is easier than ever.
5. Demand for Convenience: Consumers are increasingly looking for seamless, integrated financial solutions within the platforms they already use.
Despite these drivers, traditional credit data often falls short in assessing the creditworthiness of a large segment of the population, particularly the unbanked and informal workers. This is where alternative credit data and AI-driven models come into play.
The Importance of Alternative Credit Data
Alternative credit data includes non-traditional information such as utility payments, rent, mobile phone bills, and social media activity. This data provides a more comprehensive view of an individual’s financial behavior, particularly for those without a formal credit history. For example, regular payment of utility bills can indicate financial responsibility, while social media activity patterns can offer insights into an individual’s stability and reliability.
Accessing and integrating this alternative data is crucial for banks and fintech companies aiming to expand their customer base to underserved populations. According to a World Bank report, leveraging alternative credit data can help financial institutions better assess the creditworthiness of up to 45% of adults in Latin America who do not have a bank account, a scenario that is highly relevant to Indonesia.
Why AI-Driven Predictive Analytics?
While alternative credit data provides valuable insights, the true transformation comes from AI-driven predictive analytics. AI algorithms can analyze vast amounts of data from diverse sources, identifying patterns and correlations that human analysts might miss. These models generate comprehensive risk scores, enabling financial institutions to make more informed and accurate credit decisions.
For instance, AI-driven models can identify creditworthy individuals among underbanked and informal workers by analyzing a wide range of alternative data points. This detailed profiling allows banks and fintech companies to extend credit to a broader customer base, driving financial inclusion and expanding market reach.
Case Study: Transforming Credit Risk with AI and Alternative Data in Indonesia
Consider the case of a fintech company in Indonesia that implemented an AI-driven credit risk assessment model incorporating alternative credit data. Before adopting this approach, the company struggled to accurately assess the creditworthiness of informal workers and individuals with limited credit history. By integrating data from utility bills, mobile phone payments, and social media activity, the AI model generated detailed risk scores for these individuals.
The results were impressive. The company saw a 25% increase in approval rates for credit applications from underbanked individuals while maintaining a low default rate. This expansion not only grew their customer base but also significantly contributed to financial inclusion by providing access to credit for those previously excluded from the formal financial system.
Practical Steps for Risk Executives
For risk executives at banks and fintech companies, the path forward involves several key steps:
1. Invest in Data Integration: Ensure access to a wide range of alternative credit data sources. This can include utility companies, mobile network operators, and social media platforms.
2. Leverage AI and Machine Learning: Implement AI-driven predictive analytics models to analyze alternative credit data and generate comprehensive risk scores.
3. Focus on Financial Inclusion: Develop strategies specifically targeting underbanked and informal workers, leveraging alternative data to assess their creditworthiness accurately.
4. Stay Ahead of Regulatory Changes: Keep abreast of regulatory developments that may impact the use of alternative data and AI in credit risk assessment.
The future of credit risk management in Indonesia lies in the integration of alternative data and AI-driven predictive analytics. These technologies offer the potential to transform credit risk assessment, enabling banks and fintech companies to accurately evaluate the creditworthiness of underbanked and informal workers. As the embedded finance market continues to grow, adopting these innovative solutions will be critical for financial institutions to stay competitive and drive financial inclusion.
At 1datapipe, we understand the importance of leveraging AI-powered customer analytics to empower financial institutions. Our solutions help credit risk executives accurately assess income and accelerate customer approvals, particularly for underbanked populations and informal workers. Reach out to our team at 1datapipe to learn more about how our AI-powered analytics can benefit your organization.
Are you ready to embrace the future of credit risk management and unlock the potential of AI-powered predictive analytics?