Unlocking Financial Insights: The Critical Role of Supervised Machine Learning in Income and Fraud Analysis
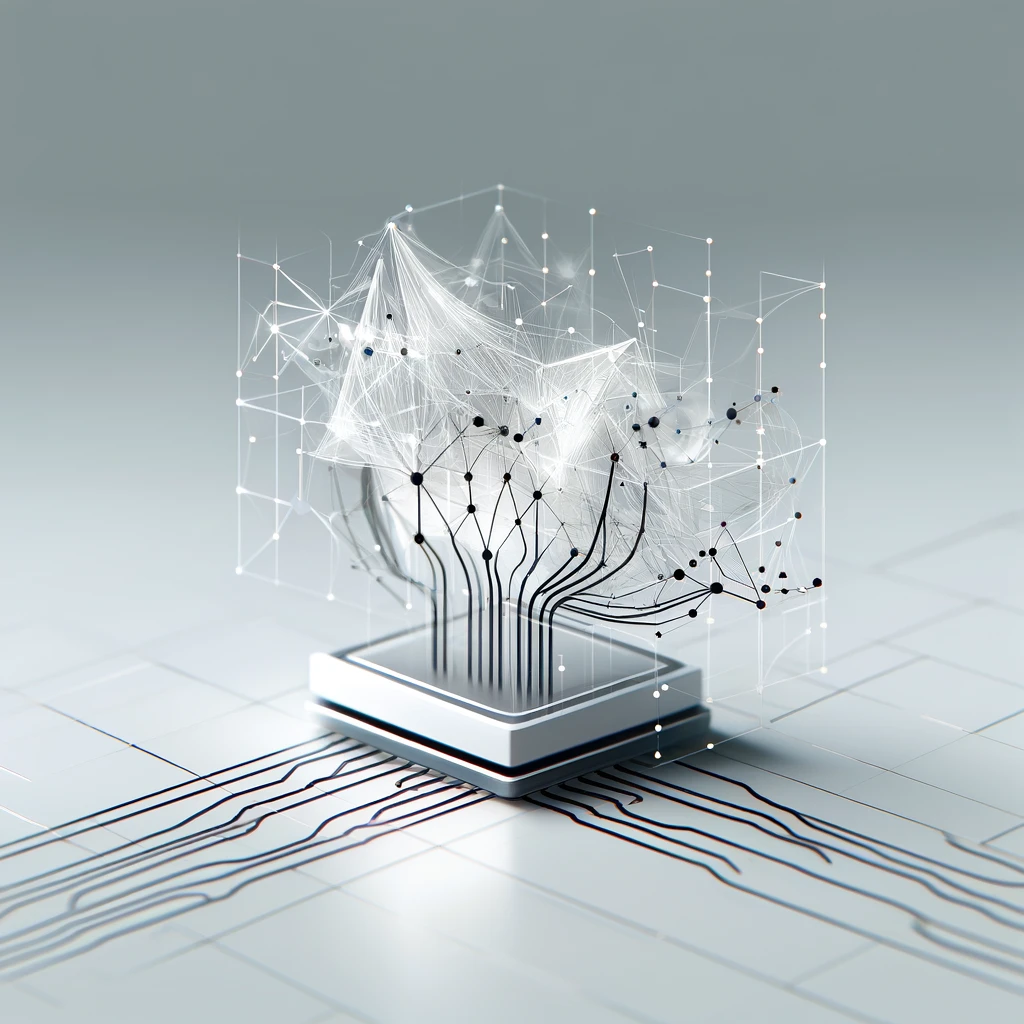
In Brazil’s rapidly evolving financial landscape, where the informal sector represents a significant portion of the economy, financial services face a daunting task: accurately assessing income and detecting fraud among a largely unbanked population. Picture a bustling market, filled with vendors whose incomes fluctuate daily. How can banks offer credit without precise data? This scenario highlights a global challenge in financial services—the need for advanced income insights and robust fraud prevention strategies to ensure financial inclusion.
The Gap in Traditional Data Collection
Traditionally, financial institutions have relied on conventional data points like credit history and formal employment records to assess a customer’s financial profile. However, this method often leaves out a large portion of the population working in informal sectors or those without extensive credit histories. According to the World Bank, informal employment makes up 27% of the global workforce, and in emerging markets like Brazil, nearly 40% of the labor force works in informal sectors. These individuals often lack access to formal financial services, making it difficult to assess their creditworthiness using traditional methods.
This gap in data collection also creates vulnerabilities that fraudsters exploit. As reliance on outdated systems persists, many financial institutions still use unsupervised machine learning models, which don’t effectively prevent fraud. These models often yield high false-positive rates, which frustrates customers and misses real fraud signals. McKinsey reported that global financial fraud costs exceeded $42 billion in 2022, with a significant portion attributable to inadequate fraud detection tools.
The Advantages of Supervised Machine Learning
Supervised machine learning offers a more refined approach to tackling these challenges. Unlike unsupervised models, supervised learning uses labeled datasets to train algorithms, enabling them to predict outcomes based on past data. This approach is particularly effective in two critical areas: income stability assessment and fraud detection.
Income Stability Assessment
Supervised learning models use a combination of traditional data (such as tax records and salary information) and alternative data points (like utility bills and rental payments) to build a more complete financial profile of an individual. This enables financial institutions to more accurately assess the income of informal workers, who are often left out of traditional credit scoring systems. By incorporating alternative data sources, supervised learning helps financial institutions extend credit to the “credit invisible”—those who lack a formal credit history but are financially stable.
For example, in Brazil’s informal sector, where gig economy workers and small business owners make up a significant portion of the workforce, leveraging alternative data like mobile phone usage and spending habits can provide a clearer picture of an individual’s financial stability.
Fraud Prevention
Fraud detection is another area where supervised machine learning excels. By training models on datasets where fraudulent behavior is labeled, these models can more accurately predict and prevent fraud. This enables financial institutions to detect subtle patterns of fraud that might otherwise go unnoticed. Supervised models can learn from historical data to identify anomalies in real-time, helping banks and financial services companies drastically reduce false positives—which not only strain resources but can also damage customer relationships.
According to Aite-Novarica, using machine learning for fraud detection can reduce false positives by up to 70%, significantly improving both operational efficiency and customer satisfaction.
Case Studies and Real-World Impact
The real-world impact of supervised machine learning in income assessment and fraud detection is backed by compelling evidence. A Javelin Strategy & Research report found that financial institutions using machine learning-based fraud detection systems experienced a 30% reduction in fraud detection errors, saving millions of dollars annually. Furthermore, these models can reduce the need for manual transaction reviews by up to 40%, leading to streamlined operations and improved service delivery.
Additionally, banks that have implemented supervised learning models for income estimation have seen 20% higher approval rates among previously underserved populations, increasing their customer base while maintaining acceptable risk levels.
1datapipe’s Role in Pioneering Customer Analytics
At 1datapipe, we’ve embraced the transformative power of supervised machine learning to enhance both income assessment and fraud detection. Our Income Estimation Insights use a wide range of alternative data sources, including utility payments, rental history, and even behavioral data from mobile phone usage, to create a more comprehensive financial profile for customers—especially those in the informal sector. This allows lenders to accurately assess income and extend credit where it was previously impossible.
On the fraud prevention side, our Secure ID & Fraud Score leverages supervised machine learning models to identify potential fraud in real-time. These models analyze past behaviors, detect anomalies, and flag transactions or applications that carry a high risk of fraud. As a result, our solution not only helps financial institutions mitigate risk but also reduces false positives, leading to faster and more accurate decision-making.
The Future of Financial Services: AI and Machine Learning
As financial institutions continue to adopt AI and machine learning technologies, the future of income estimation and fraud detection looks promising. Supervised learning models are becoming more sophisticated, with the ability to process vast amounts of data in real-time, leading to better decision-making and reduced operational costs. According to Deloitte, financial institutions that invest in AI-driven analytics can expect to see operational cost reductions of up to 25% by 2025.
The rise of machine learning also presents new opportunities for financial inclusion. In emerging markets like Brazil, where a significant portion of the population remains unbanked or underbanked, machine learning models that incorporate alternative data sources can help financial institutions reach underserved populations. By using AI to assess income and detect fraud, banks can offer more inclusive financial products, fostering economic growth and stability.
1datapipe: Leading the Charge in Financial Inclusion
As the financial services industry evolves, 1datapipe is at the forefront of leveraging AI and machine learning to drive financial inclusion and fraud prevention. Our solutions, including the Income Estimation Insights and Secure ID & Fraud Score, help financial institutions expand access to credit for the unbanked and underbanked populations, while also safeguarding against fraud.
Are you ready to redefine the boundaries of what’s possible in financial inclusion and fraud detection? Reach out to 1datapipe today to discover how our cutting-edge solutions can empower your business to serve more customers while minimizing risk.